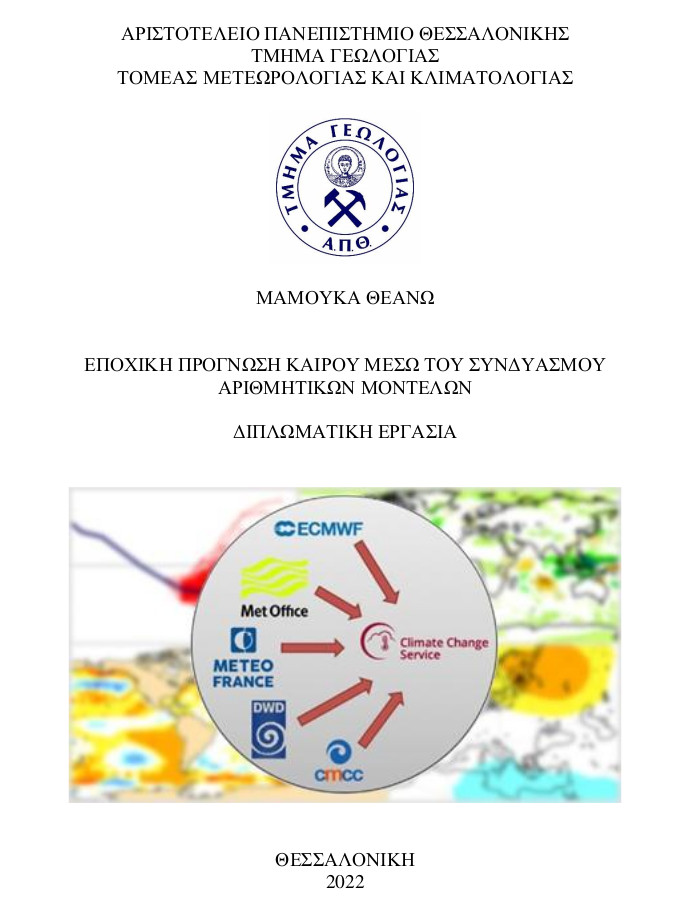
Εποχική πρόγνωση καιρού μέσω του συνδυασμού αριθμητικών μοντέλων =Seasonal weather prediction through the combination of numerical models.
Περίληψη
Η παρούσα εργασία, λοιπόν, αποσκοπεί στην αξιολόγηση των εποχικών προγνώσεων από τα έξι σημαντικότερα και πιο εύκολα προσβάσιμα, από άποψη παροχής δεδομένων, προγνωστικά κέντρα για την γεωγραφική περιοχή της Ευρώπης και την χρονική περίοδο: Μάιος-Οκτώβριος 2021.
Η μεθοδολογία που εφαρμόστηκε σε αυτό το πλαίσιο περιλαμβάνει μια αρχική επεξεργασία των δεδομένων από το κάθε κέντρο για τον υπολογισμό τεσσάρων (4) μετεωρολογικών παραμέτρων (σχετική υγρασία στα 2m, θερμοκρασία αέρα στα 2m, ταχύτητα ανέμου στα 10m και υετό) και τον συνδυασμό τους σε ένα ξεχωριστό μοντέλο. Η προγνωστική ικανότητα για κάθε μεταβλητή και κέντρο εκτιμήθηκε στη συνέχεια με βάση δύο (2) σκορ : το Mean Absolute Error (MAE) και το Fraction Skill Score (FSS).
Επιπλέον, έχει αναπτυχθεί και μία υποτυπώδης μεθοδολογία για τη δημιουργία ενός συνδυαστικού μοντέλου από τα έξι αρχικά προγνωστικά κέντρα. Σκοπός αυτού του εγχειρήματος είναι, θεωρητικά, η εξομάλυνση των σφαλμάτων από τα μεμονωμένα προγνωστικά κέντρα.
Οι μετεωρολογικές προβλέψεις που αξιοποιήθηκαν για αυτόν τον σκοπό προέρχονται από 6 προγνωστικά κέντρα : ECMWF (European Centre for Medium-Range Weather Forecasts), ΜΕΤΕΟ-FRANCE, UKMO (UK Met Office), CMCC (Euro-Mediterranean Center on Climate Change), DWD (Deutscher Wetterdienst) και NCEP (National Centers for Environmental Prediction).
Γενικά, η παράμετρος που παρουσιάζει την καλύτερη απόδοση στα προγνωστικά σκορ, για την εξεταζόμενη περίοδο, είναι η ταχύτητα ανέμου, με τη θερμοκρασία και τη σχετική υγρασία να ακολουθούν επιδεικνύοντας μέτρια έως υψηλά σφάλματα. Οι προβλέψεις για τον υετό, ωστόσο, σε όλες τις περιπτώσεις φάνηκε να πλήττονται από ιδιαίτερα χαμηλή προγνωστική ικανότητα.
Nowadays, it is generally accepted that seasonal weather forecasts hold an important role in making financial, and not only, decisions in numerous sectors of the modern society.
Therefore, the present paper aims to evaluate the seasonal weather forecasts of six of the most important and most easily accessible, data-wise, forecasting centers for the geographical area of Europe and the time period: May-October 2021.
The methodology applied in this context includes an initial processing of the data from each center to calculate four (4) meteorological parameters (relative humidity at 2m, air temperature at 2m, wind speed at 10m and precipitation) and their combination into a separate model. The predictability for each variable of every center was then assessed based on two (2) skill scores: the Mean Absolute Error (MAE) and the Fraction Skill Score (FSS).
In addition to the assessment of their predictive capacity, in the context of this work, a rudimentary methodology has been developed for the creation of a multi-model from the six initial forecasting centers. The goal of this endeavor is, in theory, to smooth the errors of the individual forecasting centers.
The forecasts used for this purpose are produced from 6 different centers: ECMWF (European Center for Medium-Range Weather Forecasts), METEO-FRANCE, UKMO (UK Met Office), CMCC (Euro-Mediterranean Center on Climate Change), DWD (Deutscher Wetterdienst) and NCEP (National Centers for Environmental Prediction).
In general, the parameter that performs the best in forecast scores for the period under consideration is wind speed, followed by temperature and relative humidity which show moderate to high errors. However, the precipitation in all cases appeared to suffer from particularly poor predictability.
Πλήρες Κείμενο:
PDFΑναφορές
Skamarock, W., Klemp, J., Dudhia, J., Gill, D., Liu, Z., Berner, J., . . . Huang, X.-Y. (2019). A Description of the Advanced Research WRF Version 4. NCAR Tech. Note NCAR/TN-556+STR,. doi:10.5065/1dfh-6p97
Easterling, D. R., Meehl, G. A., Parmesan, C., Changnon, S. A., Karl, T. R., & Mearns, L. O. (2000). Climate extremes: observations, modeling, and impacts. Science, 289(5487), 2068-2074.
Johnson, S. J., Stockdale, T. N., Ferranti, L., Balmaseda, M. A., Molteni, F., Magnusson, L., ... & Monge-Sanz, B. M. (2019). SEAS5: the new ECMWF seasonal forecast system. Geoscientific Model Development, 12(3), 1087-1117.
MacLeod, D. (2019). Seasonal forecasts of the East African long rains: insight from atmospheric relaxation experiments. Climate Dynamics, 53(7), 4505-4520.
Kilavi, M., MacLeod, D., Ambani, M., Robbins, J., Dankers, R., Graham, R., ... & Todd, M. C. (2018). Extreme rainfall and flooding over central Kenya including Nairobi city during the long-rains season 2018: causes, predictability, and potential for early warning and actions. Atmosphere, 9(12), 472.
MacLeod, D. (2018). "Seasonal predictability of onset and cessation of the east African rains." Weather and climate extremes 21, 27-35.
Chen, S. C., Wu, M. C., & Roads, J. O. (2001). Seasonal forecasts for Asia: Global model experiments. Terrestrial, Atmospheric and Oceanic Sciences, 12(2), 377-400.
Weisheimer, A., & Palmer, T. N. (2014). On the reliability of seasonal climate forecasts. Journal of the Royal Society Interface, 11(96), 20131162.
Wang, W., Chen, M., & Kumar, A. (2010). An assessment of the CFS real-time seasonal forecasts. Weather and forecasting, 25(3), 950-969.
Scoccimarro, E., Athanasiadis, P., Gualdi, S., Scaife, A. A., Bellucci, A., Hermanson, L., ... & MacLachlan, C. (2015). A Multi-System View of Wintertime NAO Seasonal Predictions. In AGU Fall Meeting Abstracts (Vol. 2015, pp. A33M-0399).
Thorncroft, C., & Pytharoulis, I. (2001). A dynamical approach to seasonal prediction of Atlantic tropical cyclone activity. Weather and forecasting, 16(6), 725-734.
Landsea, C. W., Bell, G. D., Gray, W. M., & Goldenberg, S. B. (1998). The extremely active 1995 Atlantic hurricane season: Environmental conditions and verification of seasonal forecasts. Monthly Weather Review, 126(5), 1174-1193.
Jones, C. G., & Thorncroft, C. D. (1998). The role of El Niño in Atlantic tropical cyclone activity. Weather, 53(9), 324-336.
Branković, Č., & Palmer, T. N. (2000). Seasonal skill and predictability of ECMWF PROVOST ensembles. Quarterly Journal of the Royal Meteorological Society, 126(567), 2035-2067.
Stockdale, T. N., Anderson, D. L., Alves, J. O. S., & Balmaseda, M. A. (1998). Global seasonal rainfall forecasts using a coupled ocean–atmosphere model. Nature, 392(6674), 370-373.
Batté, L., & DeÉQueÉ, M. (2011). Seasonal predictions of precipitation over Africa using coupled ocean-atmosphere general circulation models: Skill of the ENSEMBLES project multimodel ensemble forecasts. Tellus A: Dynamic Meteorology and Oceanography, 63(2), 283-299.
http://www.emy.gr/emy/el/climatology/seasonal
Bowler, N. E., Arribas, A., & Mylne, K. R. (2008). The benefits of multianalysis and poor man’s ensembles. Monthly weather review, 136(11), 4113-4129. Li, J., Swinbank, R., Grotjahn, R., & Volkert, H. (Eds.). (2016). Dynamics and predictability of large-scale, high-impact weather and climate events (Vol. 2). Cambridge University Press.
Arribas, A., Robertson, K. B., & Mylne, K. R. (2005). Test of a poor man’s ensemble prediction system for short-range probability forecasting. Monthly Weather Review, 133(7), 1825-1839.
Hoffman, R. N., & Kalnay, E. (1984). Lagged average forecasting, some operational considerations. In AIP Conference Proceedings (Vol. 106, No. 1, pp. 141-147). American Institute of Physics.
Βάρλας Γ., Κατσαφάδος Π. (2015). ΣΤΑΤΙΣΤΙΚΕΣ ΠΡΟΣΕΓΓΙΣΕΙΣ ΚΑΙ ΑΞΙΟΛΟΓΗΣΗ ΤΗΣ ΕΠΟΧΙΑΚΗΣ ΠΡΟΓΝΩΣΗΣ ΚΑΙΡΟΥ. Πρακτικά 28ου Πανελληνίου Συνεδρίου Στατιστικής.
Houtekamer, P. L., Lefaivre, L., Derome, J., Ritchie, H., & Mitchell, H. L. (1996). A system simulation approach to ensemble prediction. Monthly Weather Review, 124(6), 1225-1242.
Li, J., Swinbank, R., Grotjahn, R., & Volkert, H. (Eds.). (2016). Dynamics and predictability of large-scale, high-impact weather and climate events (Vol. 2). Cambridge University Press.
https://cds.climate.copernicus.eu/cdsapp#!/dataset/seasonal-original-single-levels?tab=overview
https://confluence.ecmwf.int/display/CKB/Description+of+the+C3S+seasonal+multi-system
https://confluence.ecmwf.int/display/CKB/Description+of+System8-v20210101+C3S+contribution
https://confluence.ecmwf.int/display/CKB/Description+of+HadGEM3-GC3.2-v20200929+C3S+contribution
https://confluence.ecmwf.int/display/CKB/Description+of+CMCC-CM2-v20191201+C3S+contribution
https://confluence.ecmwf.int/display/CKB/Description+of+GCFS2.1-v20200320+C3S+contribution
https://confluence.ecmwf.int/display/CKB/Description+of+CFSv2-v20110310+C3S+contribution
https://cds.climate.copernicus.eu/cdsapp#!/dataset/reanalysis-era5-single-levels?tab=overview
https://dtcenter.org/community-code/model-evaluation-tools-met
Wilks, D. S. (2011). Statistical methods in the atmospheric sciences (Vol. 100). Academic press. Ανακτήθηκε από εδώ : source
https://web.archive.org/web/20121002134429/http:/www.metoffice.gov.uk/media/pdf/4/4/Fact_Sheet_No._6_-_Beaufort_Scale.pdf
Thyness, V., Homleid, M., Køltzow, M., & Lien, T. (2017). Application and Verification of ECMWF Products 2017. Ανακτήθηκε από εδώ : source
Haiden, T., Janousek, M., Bidlot, J., Buizza, R., Ferranti, L., Prates, F., & Vitart, F. (2018). Evaluation of ECMWF forecasts, including the 2018 upgrade. Reading, UK: European Centre for Medium Range Weather Forecasts. Ανακτήθηκε από εδώ: source
Sangelantoni L, Ferretti R, Redaelli G. Toward a Regional-Scale Seasonal Climate Prediction System over Central Italy Based on Dynamical Downscaling. Climate. 2019; 7(10):120. https://doi.org/10.3390/cli7100120
Qiao, X., Wang, S., Schwartz, C. S., Liu, Z., & Min, J. (2020). A Method for Probability Matching Based on the Ensemble Maximum for Quantitative Precipitation Forecasts. Monthly Weather Review, 148(8), 3379-3396.
Εισερχόμενη Αναφορά
- Δεν υπάρχουν προς το παρόν εισερχόμενες αναφορές.