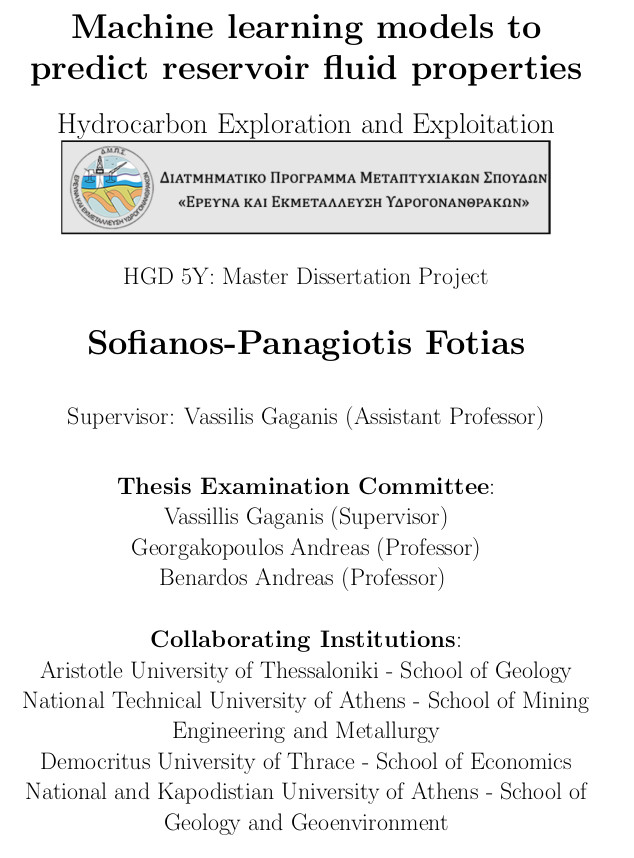
Μοντέλα μηχανικής μάθησης για την πρόβλεψη ιδιοτήτων ρευστού ταμιευτήρα = Machine learning models to predict reservoir fluid properties.
Περίληψη
The purpose of this work is the study of undersaturated reservoir oil viscosity. Starting from the bubble point pressure up to the maximum value of reservoir pressure, given that viscosity value at the bubble point is known, viscosity's change in relation to pressure needs to be predicted. In total, 17 literature correlations were evaluated and their accuracy was judged based on statistical metrics suchs as average relative error and absolute average relative error. Furhtermore, machine learning models were developed in order to create model that predct viscosity's change. Machine learning models were compared with literature correlations in a consistent way so as to be sure of the produced results. The resulting machine learning models perform better on average than even the best performing literature's correlation so the process followed in this work can be considered a success.
Πλήρες Κείμενο:
PDFΑναφορές
Abdul-Majeed, G. H., Kattan, R. R., & Salman, N. H. (1990). New correlation for estimating the viscosity of undersaturated crude oils. Journal of Canadian Petroleum Technology, 29(3).
Al-Khafaji, A. H., Abdul-Majeed, G. H., Hassoon, S. F., & others. (1987). Viscosity correlation for dead, live and undersaturated crude oils. J. Pet. Res, 6(2), 1–16.
Almehaideb, R. (1997). Improved PVT correlations for UAE crude oils. Middle East Oil Show and Conference.
API. (1997). Technical data book—petroleum refining. Metric Edition, Vols, 1(2).
Beal, C. (1946). The viscosity of air, water, natural gas, crude oil and its associated gases at oil field temperatures and pressures. Transactions of the AIME, 165(1), 94–115.
Bergman, D., & Sutton, R. P. (2006). Undersaturated oil viscosity correlation for adverse conditions. SPE Annual Technical Conference and Exhibition.
Cortes, C., & Vapnik, V. (1995). Support-vector networks. Machine Learning, 20(3), 273–297.
Cristianini, N., Shawe-Taylor, J., & others. (2000). An introduction to support vector machines and other kernel-based learning methods. Cambridge university press.
De Ghetto, G., & Villa, M. (1994). Reliability analysis on PVT correlations. European Petroleum Conference.
Dindoruk, B., & Christman, P. G. (2004). PVT properties and viscosity correlations for Gulf of Mexico oils. SPE Reservoir Evaluation & Engineering, 7(6), 427–437.
Elsharkawy, A., & Alikhan, A. (1999). Models for predicting the viscosity of Middle East crude oils. Fuel, 78(8), 891–903.
Fletcher, T. (2009). Support vector machines explained. Tutorial Paper, 1–19.
Géron, A. (2019). Hands-on machine learning with Scikit-Learn, Keras, and TensorFlow: Concepts, tools, and techniques to build intelligent systems. “ O’Reilly Media, Inc.”
Glaso, O. (1980). Generalized pressure-volume-temperature correlations. Journal of Petroleum Technology, 32(5), 785–795.
Hossain, M. S., Sarica, C., Zhang, H.-Q., Rhyne, L., & Greenhill, K. (2005). Assessment and development of heavy oil viscosity correlations. SPE International Thermal Operations and Heavy Oil Symposium.
Introduction to GP¶. (n.d.). In Introduction to GP - gplearn 0.4.2 documentation. https://gplearn.readthedocs.io/en/stable/intro.html
Kartoatmodjo, T., Schmidt, Z., & others. (1991). New correlations for crude oil physical properties. Paper SPE, 23556.
Khan, S., Al-Marhoun, M., Duffuaa, S., & Abu-Khamsin, S. (1987). Viscosity correlations for Saudi Arabian crude oils. Middle East Oil Show.
Kingma, D. P., & Ba, J. (2014). Adam: A method for stochastic optimization. ArXiv Preprint ArXiv:1412.6980.
Kouzel, B. (1965). How pressure affects liquid viscosity. Hydrocarbon Processing. March, 1965, 120.
Kulchanyavivat, S. (2005). The effective approach for predicting viscosity of saturated and undersaturated reservoir oil. Texas A&M University.
Labedi, R. M. (1982). Pvt correlations of the african crudes. 1980-1989-Mines Theses & Dissertations.
Mukherjee, H., & Brill, J. P. (1999). Multiphase flow in wells. Society of Petroleum Engineers of AIME.
Orbey, H., & Sandler, S. I. (1993). The prediction of the viscosity of liquid hydrocarbons and their mixtures as a function of temperature and pressure. The Canadian Journal of Chemical Engineering, 71(3), 437–446.
Petrosky, G. E. (1990). PVT correlations for gulf of mexico crude oils [Phdthesis]. University of Southwestern Louisiana.
Rojas, R. (1996). The backpropagation algorithm. In Neural networks (pp. 149–182). Springer.
Shawe-Taylor, J., Cristianini, N., & others. (2004). Kernel methods for pattern analysis. Cambridge university press.
Standing, M. B. (1977). Volumetric and phase behavior of oil field hydrocarbon systems. Society of petroleum engineers of AIME.
Standing, M., & Katz, D. (1981). Volumetric and phase behavior of oil hydrocarbon system. Society of Petroleum Engineers of AIME, Dallas.
Vazquez, M., & Beggs, H. (1980). Correlations for fluid physical property prediction. JPT 32 (6): 968–970 [Techreport]. SPE-6719-PA. DOI: 10.2118/6719-PA.
Εισερχόμενη Αναφορά
- Δεν υπάρχουν προς το παρόν εισερχόμενες αναφορές.