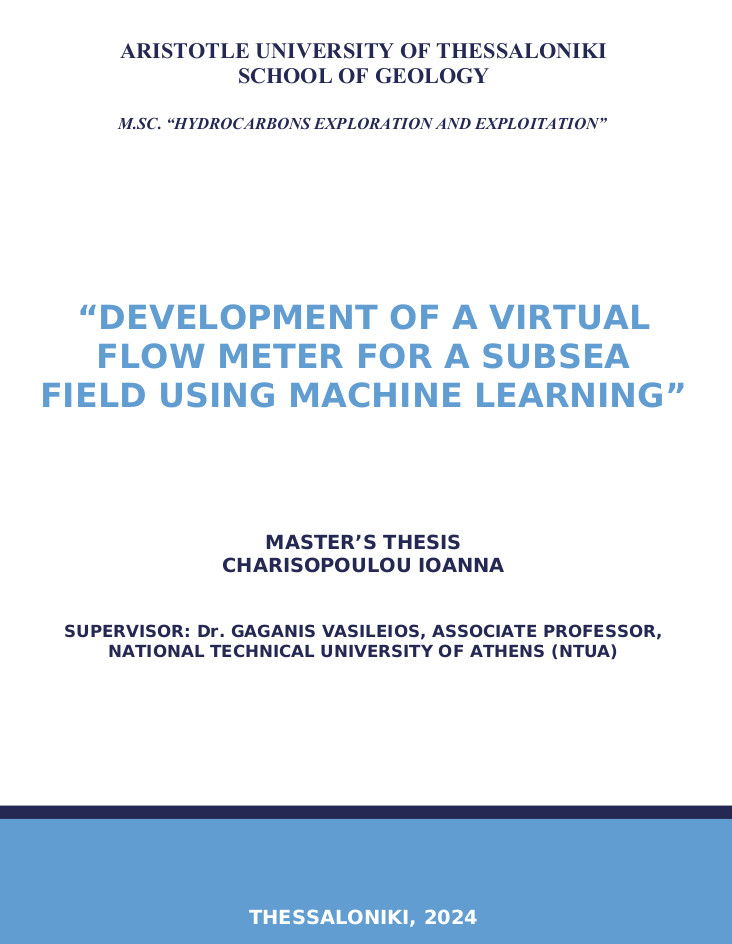
Development of a Virtual Flow Meter for a subsea field using machine learning = Ανάπτυξη εικονικού ροόμετρου σε υποθαλάσσιο δίκτυο με χρήση μηχανικής εκμάθησης.
Περίληψη
Η ακριβής μέτρηση της παραγωγής μεμονωμένων γεωτρήσεων είναι μια κρίσιμη πρόκληση στην παραγωγή υδρογονανθράκων, ειδικά σε υποθαλάσσια πεδία όπου οι παραδοσιακές μέθοδοι όπως οι test separators δεν είναι εφικτές. Στο απαιτητικό περιβάλλον των υποθαλάσσιων πεδίων υδρογονανθράκων, η χρήση test separator για την ακριβή μέτρηση της παραγωγής μεμονωμένων γεωτρήσεων μπορεί να μην είναι πάντα πρακτική επιλογή. Αυτό αποδίδεται κυρίως στην υλικοτεχνική πολυπλοκότητα που σχετίζεται με την πρόσβαση σε κεφαλές γεωτρήσεων σε μεγάλα βάθη υδάτων, την πιθανή διακοπή ροής και τα προβλήματα διάβρωσης που σχετίζονται με τους test separators. Επιπλέον, η περιορισμένη διαθεσιμότητα χώρου στον πυθμένα της θάλασσας, μαζί με την πρόκληση της διατήρησης και της βαθμονόμησης των test separators σε σημαντικά βάθη, τους καθιστούν λιγότερο ευνοϊκή επιλογή. Για την αντιμετώπιση αυτού του ζητήματος, χρησιμοποιούνται συνήθως πολυφασικά ροόμετρα (MPFMs), αλλά η εγκατάστασή τους απαιτεί πολύπλοκες υποθαλάσσιες εργασίες και συνεπάγεται σημαντικό κόστος. Μια καινοτόμος εναλλακτική λύση είναι η εφαρμογή των εικονικών πολυφασικών ροόμετρων (VFMs), όπου οι ρυθμοί ροής σε κάθε κεφαλή γεώτρησης υπολογίζονται με βάση τις ήδη διαθέσιμες μετρήσεις. Αυτή η μεταπτυχιακή εργασία διερευνά την ανάπτυξη και την εφαρμογή των VFM για την εκτίμηση της παραγωγής κάθε φάσης από διασυνδεδεμένες γεωτρήσεις σε υποθαλάσσιο πεδίο. Η έρευνα επικεντρώνεται στη δημιουργία ενός ρεαλιστικού μοντέλου δικτύου γεωτρήσεων χρησιμοποιώντας λογισμικό προσομοίωσης αγωγών για να μιμηθεί τη σύνθετη δυναμική ροής στο πεδίο. Μέσω επαναληπτικών προσομοιώσεων υπό διαφορετικές συνθήκες παραγωγής, συλλέγονται σχετικά δεδομένα για την εκπαίδευση κατάλληλων μοντέλων μηχανικής εκμάθησης. Η μελέτη δείχνει τη δυνατότητα των VFM στην ακριβή εκτίμηση της παραγωγής γεωτρήσεων συνδυάζοντας δεδομένα από μόνιμα εγκατεστημένα μανόμετρα στις κεφαλές των γεωτρήσεων με μετρήσεις συνολικής ροής που λαμβάνονται από έναν κύριο διαχωριστή. Η προτεινόμενη μαθηματική προσέγγιση επιτρέπει τον προσδιορισμό της συνεισφοράς της κάθε γεώτρησης ξεχωριστά στη συνολική παραγωγή στον διαχωριστή. Τα αποτελέσματα καταδεικνύουν την αποτελεσματικότητα των VFM στην παροχή ακριβών εκτιμήσεων της παραγωγής κάθε γεώτρησης, διευκολύνοντας τη βέλτιστη διαχείριση της και ενισχύοντας τη συνολική απόδοση παραγωγής υδρογονανθράκων. Επιπλέον, η υιοθέτηση των VFM εξαλείφει την ανάγκη για φυσικό διαχωρισμό των φάσεων ροής, μειώνοντας το λειτουργικό κόστος και την πολυπλοκότητα.
Πλήρες Κείμενο:
PDFΑναφορές
Açikgöz, M., Franca, F., & Lahey Jr, R. T. (1992). An experimental study of three-phase flow regimes. International Journal of Multiphase Flow, 18(3), 327-336.
Al-Awadi, H. (2011). Multiphase characteristics of high viscosity oil (Doctoral dissertation).
Al-Dogail, A. S., & Gajbhiye, R. N. (2021). Effects of density, viscosity and surface tension on flow regimes and pressure drop of two-phase flow in horizontal pipes. Journal of Petroleum Science and Engineering, 205, 108719.
Ali, S. F. (2009). Two-phase flow in a large diameter vertical riser (Doctoral dissertation, Cranfield University).
Al-Qutami, T. A., Ibrahim, R., & Ismail, I. (2017, September). Hybrid neural network and regression tree ensemble pruned by simulated annealing for virtual flow metering application. In 2017 IEEE International Conference on Signal and Image Processing Applications (ICSIPA) (pp. 304-309). IEEE.
Andrade, G. M., de Menezes, D. Q., Soares, R. M., Lemos, T. S., Teixeira, A. F., Ribeiro, L. D., ... & Pinto, J. C. (2022). Virtual flow metering of production flow rates of individual wells in oil and gas platforms through data reconciliation. Journal of Petroleum Science and Engineering, 208, 109772.
Andrianov, N. (2018). A machine learning approach for virtual flow metering and forecasting. IFAC-PapersOnLine, 51(8), 191-196.
Bannwart, A. C., Rodriguez, O. M. H., Trevisan, F. E., Vieira, F. F., & De Carvalho, C. H. M. (2009). Experimental investigation on liquid–liquid–gas flow: Flow patterns and pressure-gradient. Journal of petroleum science and engineering, 65(1-2), 1-13.
Barjouei, H. S., Ghorbani, H., Mohamadian, N., Wood, D. A., Davoodi, S., Moghadasi, J., & Saberi, H. (2021). Prediction performance advantages of deep machine learning algorithms for two-phase flow rates through wellhead chokes. Journal of Petroleum Exploration and Production, 11, 1233-1261.
Bello, O., Ade-Jacob, S., & Yuan, K. (2014, April). Development of hybrid intelligent system for virtual flow metering in production wells. In SPE Intelligent Energy Conference & Exhibition. OnePetro.
Berry, M. W., Mohamed, A., & Yap, B. W. (Eds.). (2019). Supervised and unsupervised learning for data science. Springer Nature.
Bikmukhametov, T., & Jäschke, J. (2020). First principles and machine learning virtual flow metering: a literature review. Journal of Petroleum Science and
Engineering, 184, 106487.
Darzi, M., & Park, C. (2017, November). Experimental visualization and numerical simulation of liquid-gas two-phase flows in a horizontal pipe. In ASME International Mechanical Engineering Congress and Exposition (Vol. 58424, p. V007T09A011). American Society of Mechanical Engineers.
Dong, F., Liu, X., Deng, X., Xu, L., & Xu, L. A. (2001). Identification of two-phase flow regimes in horizontal, inclined and vertical pipes. Measurement Science and Technology, 12(8), 1069.
Falcone, G., Hewitt, G. F., Alimonti, C., & Harrison, B. (2001, September). Multiphase flow metering: current trends and future developments. In SPE annual technical conference and exhibition. OnePetro.
Gryzlov, A., Mironova, L., Safonov, S., & Arsalan, M. (2021, October). Evaluation of machine learning methods for prediction of multiphase production rates. In SPE Symposium: Artificial Intelligence-Towards a Resilient and Efficient Energy Industry. OnePetro.
Hibiki, T., & Tsukamoto, N. (2023). Drift-flux model for upward dispersed two-phase flows in vertical medium-to-large round tubes. Progress in Nuclear Energy, 158, 104611.
Ibrahim, A. F., Al-Dhaif, R., Elkatatny, S., & Al Shehri, D. (2022). Machine Learning Applications to Predict Surface Oil Rates for High Gas Oil Ratio Reservoirs. Journal of Energy Resources Technology, 144(1).
Ishak, M. A. B., Ismail, I. B., & Al-Qutami, T. A. H. (2021, July). Data Driven Versus Transient Multiphase Flow Simulator for Virtual Flow Meter Application. In 2020 8th International Conference on Intelligent and Advanced Systems (ICIAS) (pp. 1-4). IEEE.
Ishak, M. A., Al-qutami, T. A. H., & Ismail, I. Virtual Multiphase Flow Meter using combination of Ensemble Learning and first principle physics based. International Journal on Smart Sensing and Intelligent Systems, 15(1), 1-21.
Ishak, M. A., Hasan, A. Q., Aziz, T., Ellingsen, H., Ruden, T., & Khaledi, H. (2020, October). Evaluation of Data Driven Versus Multiphase Transient Flow Simulator for Virtual Flow Meter Application. In Offshore Technology Conference Asia. OnePetro.
Jafarizadeh, F., Rajabi, M., Tabasi, S., Seyedkamali, R., Davoodi, S., Ghorbani, H., ... & Csaba, M. (2022). Data driven models to predict pore pressure using
drilling and petrophysical data. Energy Reports, 8, 6551-6562.
Javaid, A., Mohammed, A., & Ghaithan, A. (2022). A regression-based model for prediction of flowmeters calibration cost in oil and gas industry. Flow Measurement and Instrumentation, 86, 102191.
Knotek, S., Fiebach, A., & Schmelter, S. (2016, September). Numerical simulation of multiphase flows in large horizontal pipes. In Proceedings of the 17th International Flow Measurement Conference FLOMEKO.
Kotsiantis, S. B., Zaharakis, I., & Pintelas, P. (2007). Supervised machine learning: A review of classification techniques. Emerging artificial intelligence applications in computer engineering, 160(1), 3-24.
Lee, J. Y. , Ishii, M., and Kim, N. S. , 2008, “Instantaneous and Objective Flow Regime Identification Method for the Vertical Upward and Downward Co-Current Two-Phase Flow,” Int. J. Heat Mass Transfer, 51, pp. 3442–3459.
Liu, M., Kim, G., Bauckhage, K., & Geimer, M. (2022). Data-Driven Virtual Flow Rate Sensor Development for Leakage Monitoring at the Cradle Bearing in an Axial Piston Pump. Energies, 15(17), 6115.
Lv, X., Zhang, D., Song, G., Su, G., Tian, W., & Qiu, S. (2023). Experimental study on flow patterns in narrow rectangular channels. Progress in Nuclear Energy, 156, 104562.
Ma, Y., Li, C., Pan, Y., Hao, Y., Huang, S., Cui, Y., & Han, W. (2021). A flow rate measurement method for horizontal oil-gas-water three-phase flows based on
Venturi meter, blind tee, and gamma-ray attenuation. Flow Measurement and Instrumentation, 80, 101965.
Manami, M., Seddighi, S., & Örlü, R. (2023). Deep learning models for improved accuracy of a multiphase flowmeter. Measurement, 206, 112254.
Mekisso, H. M. (2013). Comparison of frictional pressure drop correlations for isothermal two-phase horizontal flow. Oklahoma State University.
Mercante, R., & Netto, T. A. (2022). Virtual flow predictor using deep neural networks. Journal of Petroleum Science and Engineering, 213, 110338.
Mohammed, S. K., Hasan, A. H., Ibrahim, A., Dimitrakis, G., & Azzopardi, B. J. (2019). Dynamics of flow transitions from bubbly to churn flow in high viscosity oils and large diameter columns. International Journal of Multiphase Flow, 120, 103095.
Mohammed, S. K., Hasan, A., Dimitrakis, G., & Azzopardi, B. J. (2018). Churn flow in high viscosity oils and large diameter columns. International Journal of Multiphase Flow, 100, 16-29.
Montoya, G., Lucas, D., Baglietto, E., & Liao, Y. (2016). A review on mechanisms and models for the churn-turbulent flow regime. Chemical Engineering Science, 141, 86-103.
Mu, J., McArdle, S., Ouyang, J., & Wu, H. (2023). Single well virtual metering research and application based on hybrid modeling of machine learning and mechanism model. Journal of Pipeline Science and Engineering, 100111.
Mukherjee, H., & Brill, J. P. (1999). Multiphase flow in wells. Memorial Fund of AIME, Society of Petroleum Engineers Inc.: Richardson, TX, USA.
Mukherjee, T., Das, G., & Ray, S. (2014). Sensor‐based flow pattern detection—gas–liquid–liquid upflow through a vertical pipe. AIChE Journal, 60(9), 3362-3375.
Naidek, B. P., Kashiwakura, L. Y., Alves, R. F., Bassani, C. L., Stel, H., & Morales, R. E. (2017). Experimental analysis of horizontal liquid-gas slug flow pressure drop in d-type corrugated pipes. Experimental Thermal and Fluid Science, 81, 234-243.
Najmi, K., McLaury, B. S., Shirazi, S. A., & Cremaschi, S. (2015). Experimental study of low concentration sand transport in wet gas flow regime in horizontal pipes. Journal of Natural Gas Science and Engineering, 24, 80-88.
Oyelade, A. O., & Oyediran, A. A. (2021). Nonlinear dynamics of horizontal pipes conveying two phase flow. European Journal of Mechanics-A/Solids, 90, 104367.
Pagan, E., Williams, W. C., Kam, S., & Waltrich, P. J. (2017). A simplified model for churn and annular flow regimes in small-and large-diameter pipes. Chemical Engineering Science, 162, 309-321.
Perez, V. H. (2007). Gas-liquid two-phase flow in inclined pipes. University of Nottingham: Nottingham, UK.
Quintino, A. M., da Rocha, D. L. L. N., Fonseca Júnior, R., & Rodriguez, O. M. H. (2021). Flow pattern transition in pipes using data-driven and physics-informed
machine learning. Journal of Fluids Engineering, 143(3).
Roxas II, R., Evangelista, M. A., Sombillo, J. A., Nnabuife, S. G., & Pilario, K. E. (2022). Machine Learning Based Flow Regime Identification using Ultrasonic Doppler Data and Feature Relevance Determination. Digital Chemical Engineering, 3, 100024.
Schmid, D., Verlaat, B., Petagna, P., Revellin, R., & Schiffmann, J. (2022). Flow pattern observations and flow pattern map for adiabatic two-phase flow of carbon dioxide in vertical upward and downward direction. Experimental Thermal and Fluid Science, 131, 110526.
Shaban, H., & Tavoularis, S. (2014). Measurement of gas and liquid flow rates in two-phase pipe flows by the application of machine learning techniques to differential pressure signals. International journal of multiphase flow, 67, 106-117.
Song, S., Wu, M., Qi, J., Wu, H., Kang, Q., Shi, B., ... & Gong, J. (2022). An intelligent data-driven model for virtual flow meters in oil and gas development. Chemical Engineering Research and Design, 186, 398-406.
Spedding, P. L., Donnelly, G. F., & Cole, J. S. (2005). Three phase oil-water-gas horizontal co-current flow: I. Experimental and regime map. Chemical Engineering Research and Design, 83(4), 401-411.
Thome, J. R., & Cioncolini, A. (2016). Two-phase flow pattern maps for microchannels. In Encyclopedia of Two-Phase Heat Transfer and Flow I: Fundamentals and Methods (pp. 47-84).
Thome, J. R., & Cioncolini, A. (2017). Flow boiling in microchannels. In Advances in Heat Transfer (Vol. 49, pp. 157-224). Elsevier.
Van der Meulen, G. P. (2012). Churn-annular gas-liquid flows in large diameter vertical pipes (Doctoral dissertation, University of Nottingham).
Vanvik, T., Henriksson, J., Yang, Z., & Weisz, G. (2022, October). Virtual flow metering for continuous real-time production monitoring of unconventional wells. In
Unconventional Resources Technology Conference, 20–22 June 2022 (pp. 739-746). Unconventional Resources Technology Conference (URTeC).
Wambsganss, M. W., Jendrzejczyk, J. A., & France, D. M. (1991). Two-phase flow patterns and transitions in a small, horizontal, rectangular channel. International journal of multiphase flow, 17(3), 327-342.
Woods, G. S., Spedding, P. L., Watterson, J. K., & Raghunathan, R. S. (1998). Three-phase oil/water/air vertical flow. Chemical Engineering Research and Design, 76(5), 571-584.
Xu, Q., Wang, X., Luo, X., Tang, X., Yu, H., Li, W., & Guo, L. (2022). Machine learning identification of multiphase flow regimes in a long pipeline-riser system. Flow Measurement and Instrumentation, 88, 102233.
Xu, X. X. (2007). Study on oil–water two-phase flow in horizontal pipelines. Journal of Petroleum Science and Engineering, 59(1-2), 43-58.
Yang, K., Liu, J., Wang, M., Wang, H., & Xiao, Q. (2023). Identifying flow patterns in a narrow channel via feature extraction of conductivity measurements with a support vector machine. Sensors, 23(4), 1907.
Yang, Y., Guo, J., Ren, B., Zhang, S., Xiong, R., Zhang, D., ... & Fu, S. (2019). Oil-Water flow patterns, holdups and frictional pressure gradients in a vertical pipe under high temperature/pressure conditions. Experimental Thermal and Fluid Science, 100, 271-291.
Zimmer, M. D., & Bolotnov, I. A. (2019). Slug-to-churn vertical two-phase flow regime transition study using an interface tracking approach. International Journal of Multiphase Flow, 115, 196-206.
Εισερχόμενη Αναφορά
- Δεν υπάρχουν προς το παρόν εισερχόμενες αναφορές.